Table Of Content

As the 2k design is primarily used to screen factors/variables, often a very large number of experimental units are required to complete even one full replication. For an example, 26 design with six variables requires 64 experimental units to complete one full replication. In the 2k design of experiment, blocking technique is used when enough homogenous experimental units are not available.
4 Outlook: Multiple Block Factors
Although the sex of the patient is not the main focus of the experiment—the effect of the drug is—it is possible that the sex of the individual will affect the amount of weight lost. Contrast analysis is based on either aov() or lmer() for estimating the linear model, and estimated marginal means from emmeans(), where results from aov() are based exclusively on the intra-block information and can differ from those based on lmer(). In two-stage experimentation, it is recommended that a block effect is included in the model to capture a possible shift in the mean response between the stages. In this paper, it is investigated how the inclusion of a block effect in the model affects the design and analysis of the experiment. I think most of the time it’s just a matter of convention, likely proper to each field.
Improving reproducibility in animal research by splitting the study population into several 'mini-experiments' Scientific ... - Nature.com
Improving reproducibility in animal research by splitting the study population into several 'mini-experiments' Scientific ....
Posted: Tue, 06 Oct 2020 07:00:00 GMT [source]
Complete Block Designs
When the experiment consists of multiple batches,they will undergothe same experimental protocol at different points in time and/orspace. Every step of the protocol may then introduce variation thatis specific for each batch. If samples are moved across batches betweenprocessing steps, each processing step has its own specific sample-to-batchallocation. Then, each processing step will have its own batch effect,and each of these will have to be estimated, unnecessarily increasingthe complexity of the model.
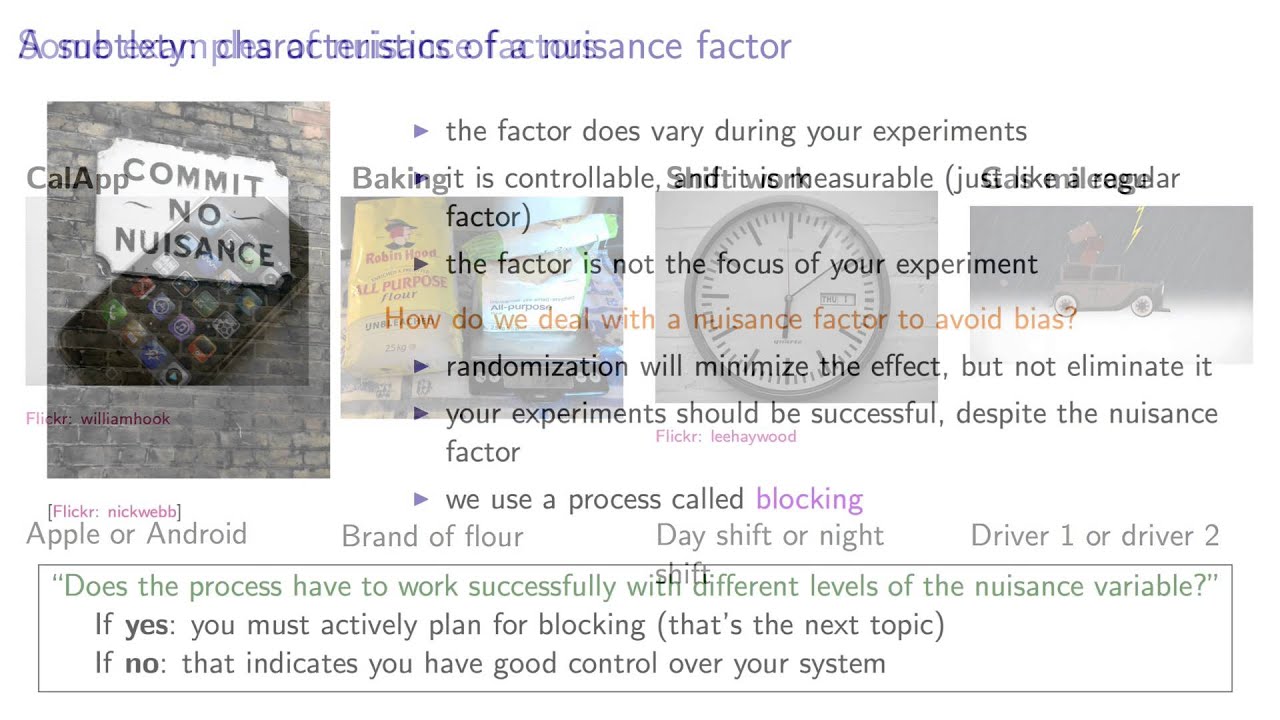
Block a few of the most important nuisance factors
Whenever, you have more than one blocking factor a Latin square design will allow you to remove the variation for these two sources from the error variation. So, consider we had a plot of land, we might have blocked it in columns and rows, i.e. each row is a level of the row factor, and each column is a level of the column factor. We can remove the variation from our measured response in both directions if we consider both rows and columns as factors in our design. If the structure were a completely randomized experiment (CRD) that we discussed in lesson 3, we would assign the tips to a random piece of metal for each test. In this case, the test specimens would be considered a source of nuisance variability. If we conduct this as a blocked experiment, we would assign all four tips to the same test specimen, randomly assigned to be tested on a different location on the specimen.
When the groups do not have a small common divisor, one can createblocks of different sizes. For example, in a nine vs ten setting,one would make eight blocks consisting of one subject of each group,and one block with the remaining three subjects (Figure Figure33B). In an experiment with multipletreatment levels, e.g., Placebo, Treatment1, and Treatment 2, the blocks would consistof subjects from all treatments. As previously, the blocks are putin random order, the order of the treatments within the blocks ischosen randomly for each block, and subjects are finally randomlyallocated according to their characteristics. A nuisance factor is a factor that has some effect on the response, but is of no interest to the experimenter; however, the variability it transmits to the response needs to be minimized or explained.
If different processing steps of the protocol have differentsizeconstraints, i.e., one step requires more batches than another, beingable to combine the smaller batches into larger batches without havingto split the smaller batches is ideal. For example, when one experimentalstep can process 12 samples at once, while another step can process24 samples at once, two batches from the first step can be combinedfor the second processing step. When this is not possible, it makesmost sense to set up the batches according to the smallest constraints,and keep these batches throughout. In the following wewill assume the conceptually simplest settingof label-free quantification without the use of reference samples.The concepts and considerations are however generally independentof the experimental setup.
Or do blocks 3 and 4 have a higher mean because they contain both compounds 3 and 4? The design cannot help us entirely disentangle the impact of blocks and treatments19. In our modelling, we will assume variation should first be described by blocks (which are generally fixed aspects of the experiment) and then treatments (which are more directly under the experimenter’s control). Here, each block has size 4, which is equal to the number of treatments in the experiment, and each treatment is applied in each block. The design is balanced having the effect that our usual estimators andsums of squares are “working.” In R, we would use the model formulay ~ Block1 + Block2 + Treat. We cannot fit a more complex model, includinginteraction effects, here because we do not have the corresponding replicates.
Create your experimental design with a simple Python command by Tirthajyoti Sarkar - Towards Data Science
Create your experimental design with a simple Python command by Tirthajyoti Sarkar.
Posted: Wed, 04 Jul 2018 02:31:34 GMT [source]
Crossover Design Balanced for Carryover Effects
If we want to analyze data from an RCBD, we need to assume that the block-by-treatment interaction is negligible. We can then merge the interaction and residual factors and use the sum of their variation for estimating the residual variance (Fig. 7.2D). Latin Square Designs are probably not used as much as they should be - they are very efficient designs. In other words, these designs are used to simultaneously control (or eliminate) two sources of nuisance variability. For instance, if you had a plot of land the fertility of this land might change in both directions, North -- South and East -- West due to soil or moisture gradients.
It is instead recommended to keep thesame batches throughout the experiment, so that possible batch effectsfrom different processing steps are combined into one overall batcheffect. We consider an example which is adapted from Venables and Ripley (2002), the original source isYates (1935) (we will see the full data set in Section 7.3). Atsix different locations (factor block), three plots of land were available.Three varieties of oat (factor variety with levels Golden.rain, Marvellousand Victory) were randomized to them, individually per location. So far we have discussed experimental designs with fixed factors, that is, the levels of the factors are fixed and constrained to some specific values. In some cases, the levels of the factors are selected at random from a larger population. In this case, the inference made on the significance of the factor can be extended to the whole population but the factor effects are treated as contributions to variance.
In an RCBD, we can estimate any treatment contrast and all effects independently within each block, and then average over blocks. We can use the same intra-block analysis for a BIBD by estimating contrasts and effects based on those blocks that contain sufficient information and averaging over these blocks. Deciphering the association between sample characteristics, e.g.,tumor types, and the proteome holds the key for improved diagnosticand treatment of diseases.
The Design Structure has one factor (oven run, Run), and the Treatment Structure two factors (Recipe and Temperature). Because every run has to be a single (nominal) temperature, Temperature and Run must occur at the same level of the experimental design. Often, the researcher is not interested in the block effect per se, but he only wants to account for the variability in response between blocks. Finally, if you expect the 'treatment effect' to differ from block to block, then interactions should be considered.
For that reason, we will start off our discussion of blocking by focusing on the main goal of blocking and leave the specific implementation details for later. In this article we tell you everything you need to know about blocking in experimental design. After that, we discuss when you should use blocking in your experimental design. Finally, we walk through the steps that you need to take in order to implement blocking in your own experimental design. In this case treatment (1) and treatment ab will be in the first block, and treatment a and treatment b will be in the second block. Blocking designs are also important in animal experiments (Lazic and Essioux 2013; Festing 2014), and replicating pre-clinical experiments in at least two laboratories can greatly increase reproducibility (Karp 2018).
They have four different dosages they want to try and enough experimental wafers from the same lot to run three wafers at each of the dosages.
The “true”protein abundance for each patient is plotted in Figure Figure11B and F for the ordered andcomplete randomized allocations, respectively. Note that, apart fromthe order of the samples, these figures are exactly the same. Randomizationis used in experimental design to reduce the prevalenceof unanticipated confounders.
No comments:
Post a Comment